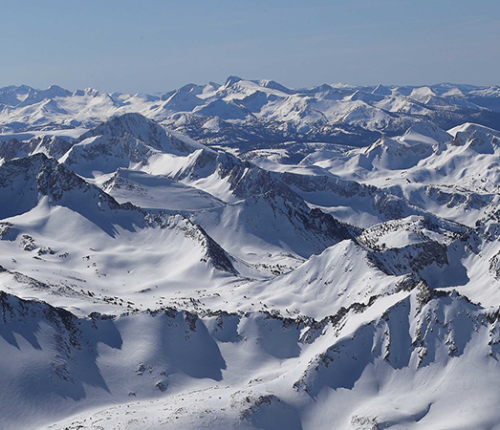
Solving the Snow Problem with Remotely Sensed Data
Aiming to develop freely available global data products that use remote sensing and machine learning to accurately estimate global snow water equivalent in near real-time
The Problem
Snow plays a critical role in storing and supplying fresh water to billions of people worldwide. In addition to the western US, it accounts for a significant portion of our water resources in areas like the Ganges and Indus River basins. However, the amount of snow can vary greatly from year to year and season to season, and this variability is only worsened by climate change. Too much water from snow melt, too fast, and people die from floods. Too little without foresight, and crops fail and people perish.
One of the holy grail challenges in snow science has been accurately measuring the volume of water in snow, snow water equivalent (SWE), in real-time, using low-cost satellite imaging. Current methods can accurately measure snow water equivalent, but they are extremely expensive, complicated, and time-consuming - and thus are accessible only to wealthiest water constituents. Furthermore, these computations arrive on a delayed timeline that precludes their use in seasonal management decisions. As a result, water managers in most of the world have limited information to make critical decisions.
The Opportunity
We see an exciting opportunity to create an AI-powered scalable data product that leverages freely available global satellite datasets, rather than expensive and limited instruments, and to develop a web-based platform that puts free, life-saving snow and water data in the hands of anyone. This advancement has the potential to significantly improve the reliability of fresh water system forecasting, helping billions of people better prepare for both floods and droughts.
Our Vision
Schmidt DSE aims to develop freely available global data products that use remote sensing and machine learning to accurately estimate global snow water equivalent in near real-time. Partnered with lead scientists in the field, including UC Berkeley’s Dr. Manuela Girotto, we seek to leverage and improve current approaches to make a dynamic model that can be applied globally with little resource use and with available data.
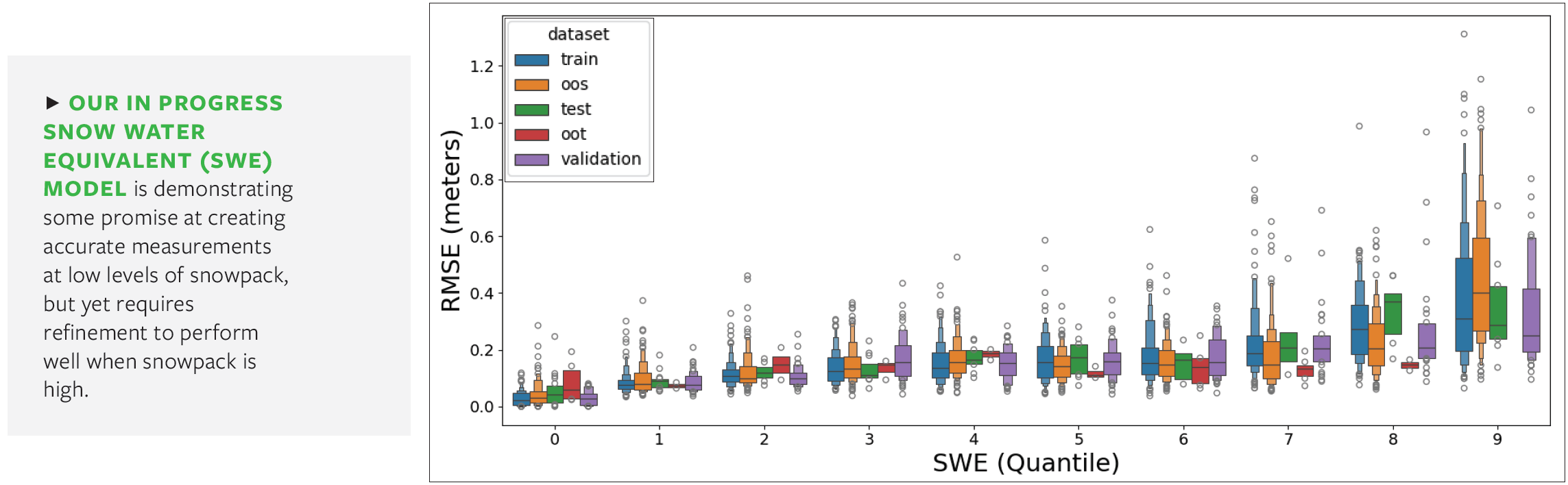
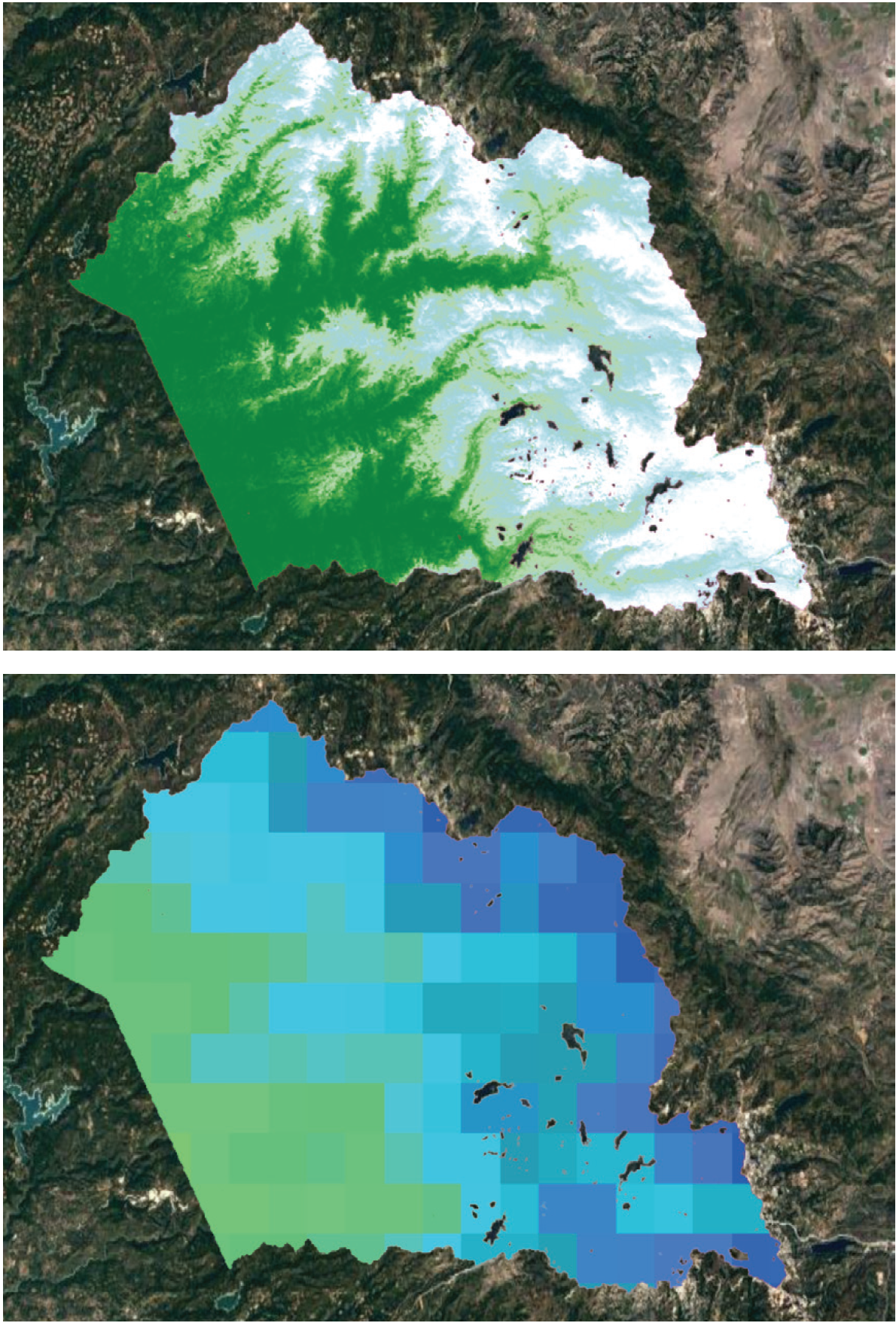
Where We Are Now
Building a Novel Model: In collaboration with leading researchers at Berkeley and beyond, we are refining our snow water equivalent model using satellite-obtained Sentinel radar data to enhance its accuracy, aiming to create a model that utilizes globally available data, is not hindered by cloud cover, is cost-effective, and maintains or improves precision of current model, at a finer geographic scale. While the development of novel modeling approaches is time-consuming and carries some level of risk, we firmly believe that the potential for far-reaching impact justifies the risks involved in this project.
Leveraging a Dynamic Data Pipeline: Furthermore, the data pipeline we're building in this project has been invaluable to our other AI-related initiatives, including vegetation estimation for our fire recovery project in partnership with the National Park Service (NPS) and cover crop estimation in our regenerative agriculture work.
Where We Are Going
Model Status: Our modeling effort for estimating snow water equivalent has improved upon the accuracy and spatial resolution of existing models in the field. Despite these encouraging results, our current prototype has challenges generating accurate outputs during conditions when snowpack is at its highest. We are continuing to iterate our modeling process to further improve the accuracy and utility over prior versions.
Feedback from On-the-Ground Water Managers: We are actively building relationships with international stakeholders to ensure the practical utility of our model on the ground. As our model becomes more mature, we will expand our work with these stakeholders to develop the web based tools that they can use to easily access the data and put it to work in meaningful ways. These efforts to connect our preliminary model outputs with water managers will be a defining area of focus for our next quarter of work.